With its roots primarily in quantitative systems pharmacology (QSP), often originating from mathematical oncology, virtual populations represent emerging mathematical modeling techniques.
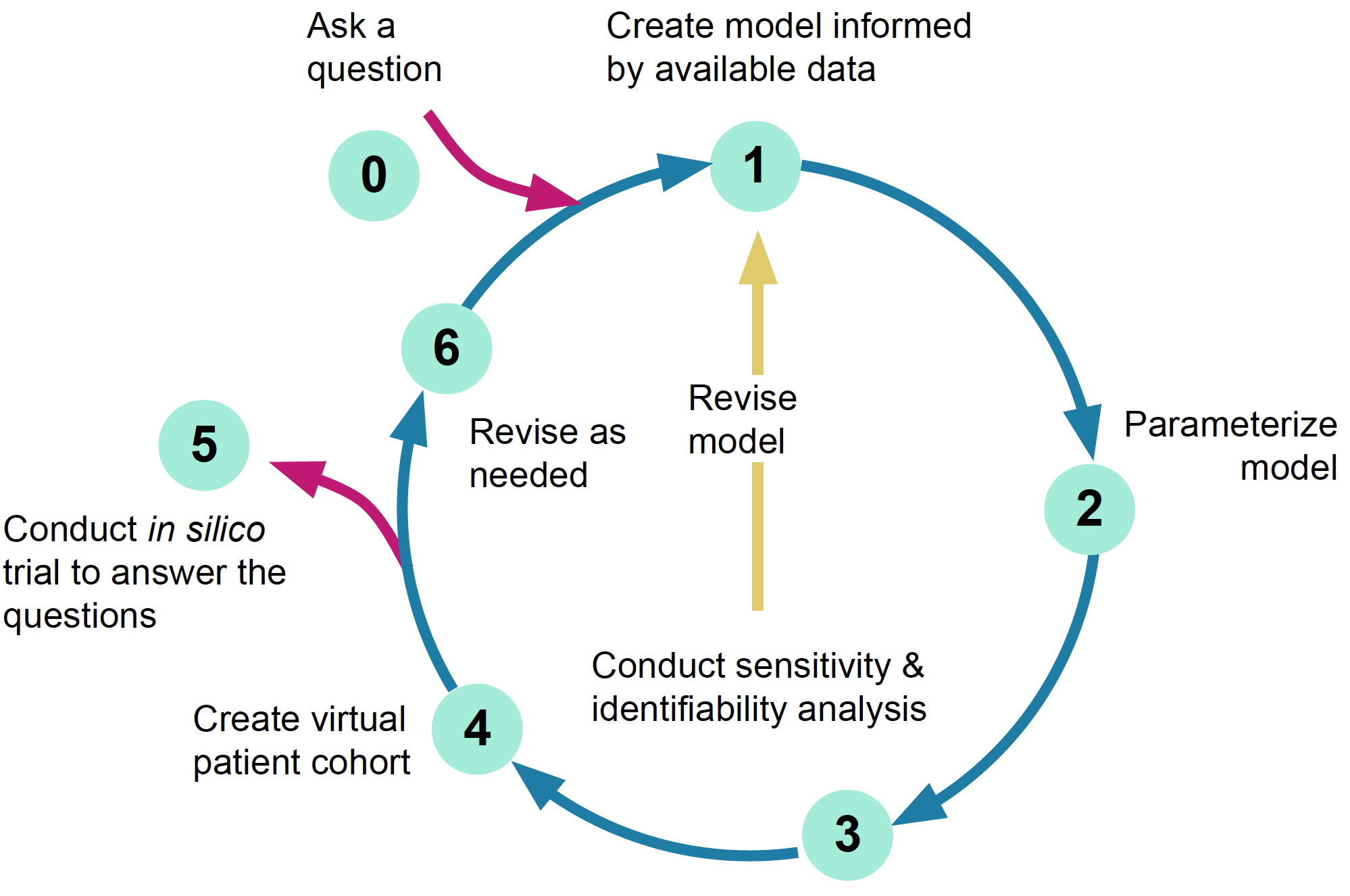
BACK: Models & AI

Example
Step 0: Ask a question (simplified)
What is the effects size (Cohen’s d value) of a perfect mechanism of action (MOA) that addresses the root cause of weekend migraine and how does the effect size depend on typical migraine phenotypes in a small sample?
Context: Many migraine patients experience weekend migraine.
Question informs RCT
- Hypothesis Generation: Intervention effectiveness and influencing factors.
- Optimal Study Design: Determine RCT design elements such as sample size and participant stratification.
- Identification of Subpopulations: Discover which patient subgroups may benefit most from the intervention.
Questions does not provide information on
- Outcome Expectations: Set realistic expectations for RCT outcomes based on in silico trial data.
- Dose Optimization: Explore different dosing strategies to refine the RCT treatment protocol.
- Clinical risk: Identify patients at higher risk of developing MOA-induced abnormalities, toxicity or adverse side effects
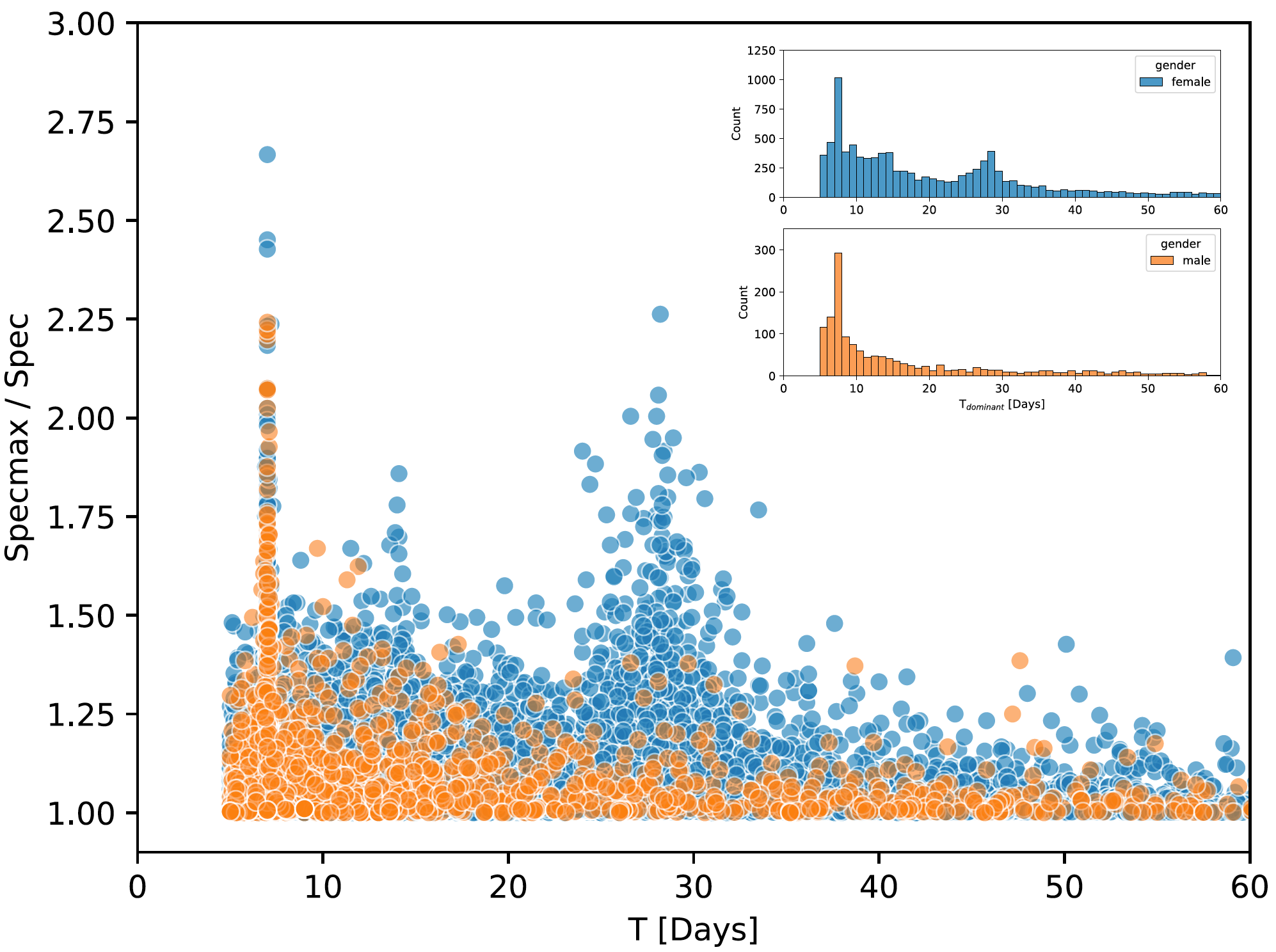
Step 2: Parameterize model

Step 3: Conduct sensitivity & identifiability analysis
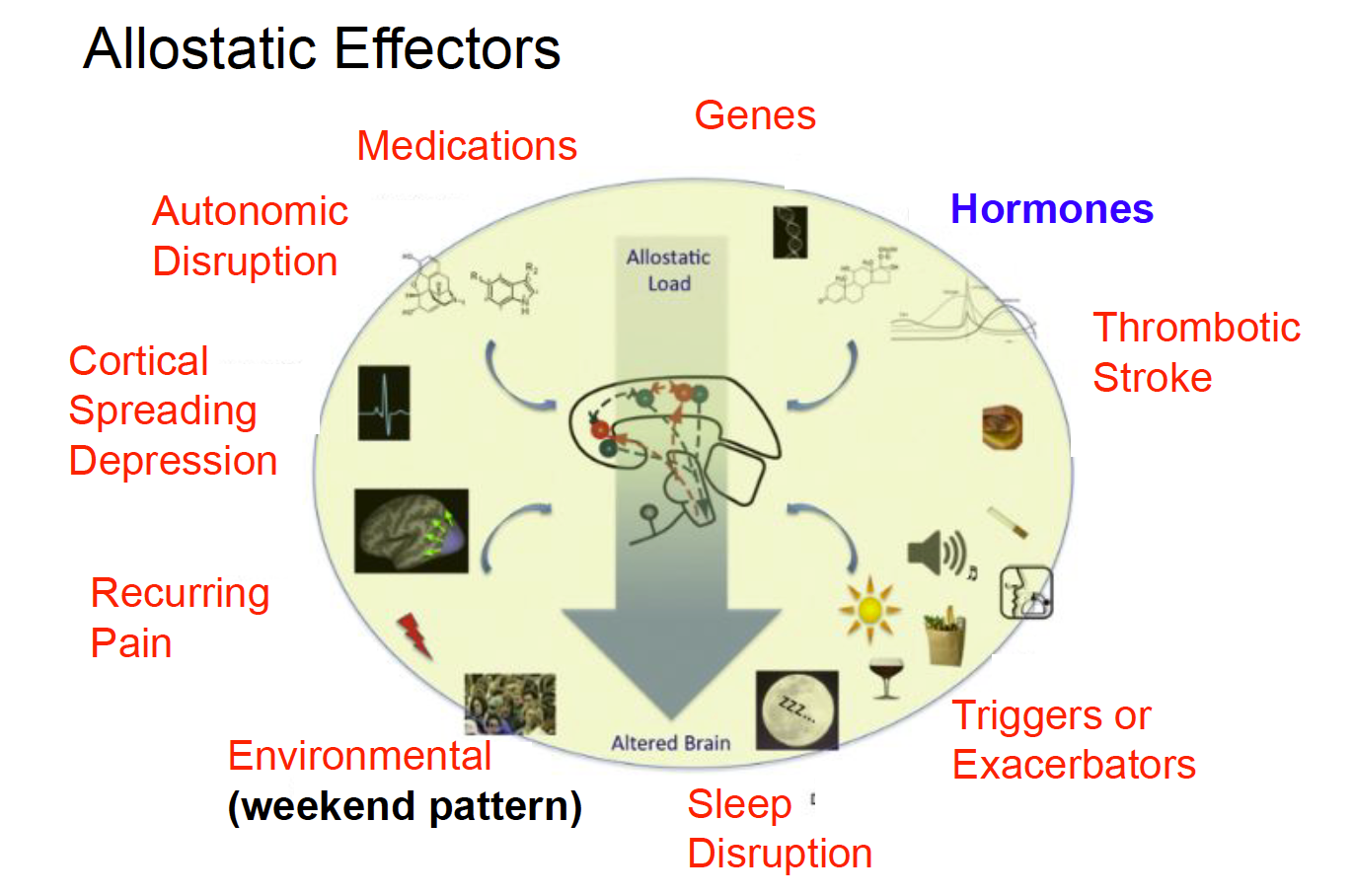
Step 4: Create virtual patient cohorts
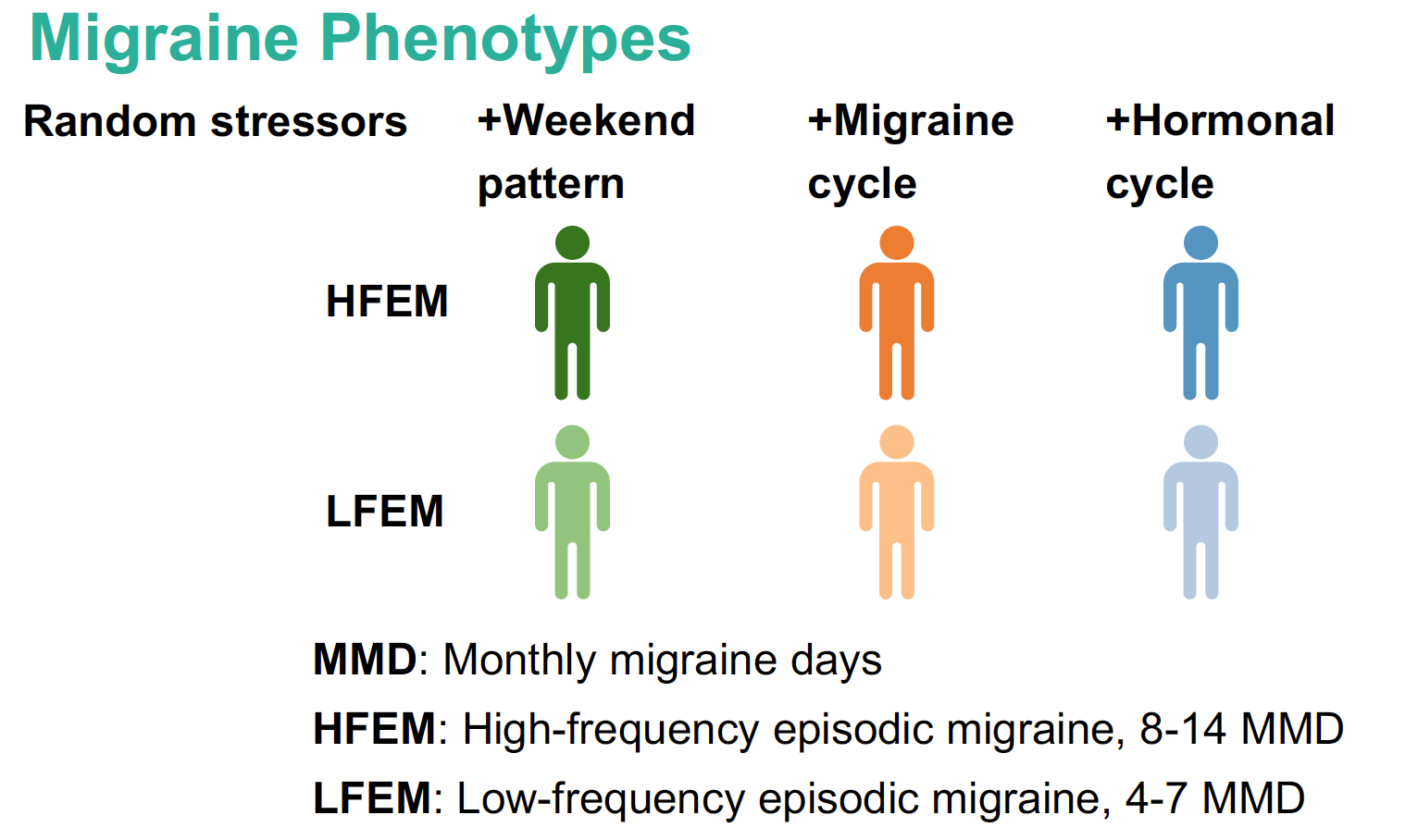
Step 5: Conduct in silico trial to answer the questions
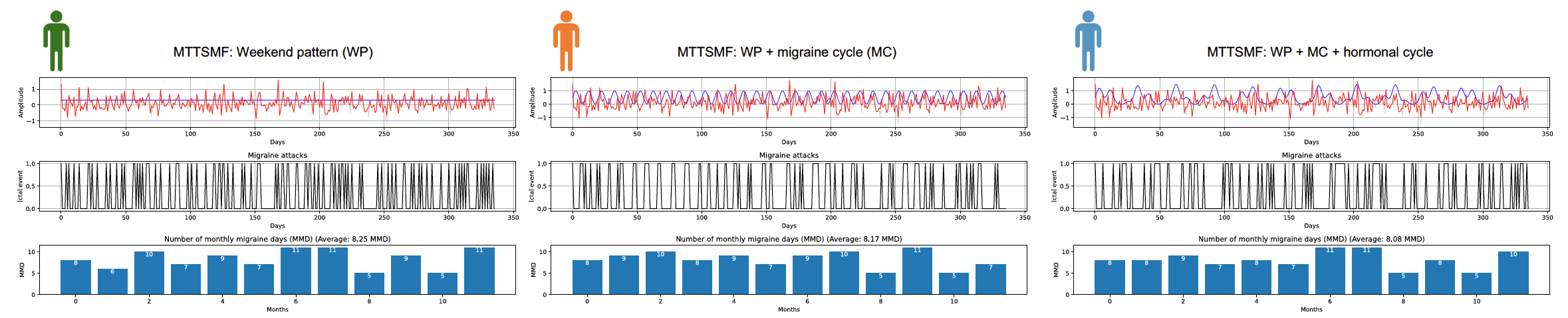
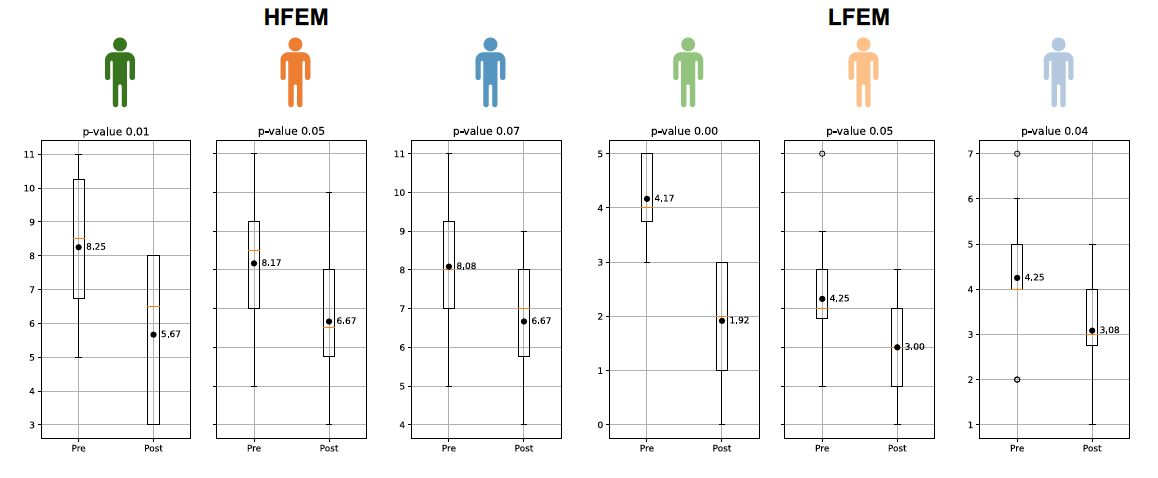
